Bias in clinical algorithms make health disparities worse
When doctors make diagnoses and recommend treatments for patients, they believe they are giving people of all races and ethnicities equal treatment. But because of bias that exists in many clinical algorithms, doctors are unintentionally giving people of color worse treatment, write the authors of a recent article in the New England Journal of Medicine.
A clinical algorithm is a fancy term for a flow chart that doctors use to help them diagnose patients and make treatment decisions based on patient risk (see the image below for an example of an algorithm).
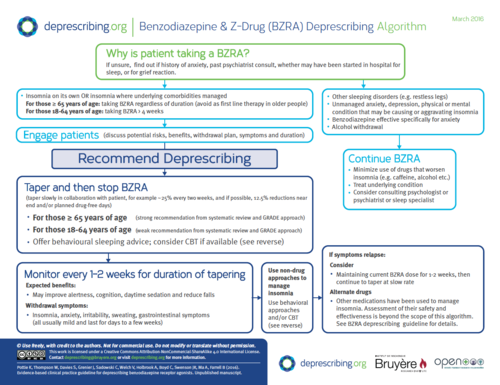
In the NEJM piece, Dr. Darshali Vyas at Massachusetts General Hospital, Dr. Leo Eisenstein at NYU Langone Hospital, and Dr. David Jones at Harvard Medical School show how, through these clinical algorithms, race has been “subtly inserted” into the decisions that doctors make, often without them realizing.
Adjustments to algorithms based on race “risk baking inequity into the system.”
Here are some examples of how clinical algorithms incorporate race in ways that exacerbate health disparities, as outlined by Vyas et al.:
- The heart failure risk score written by the American Heart Association ranks Black patients having a lower risk of dying in the hospital compared to non-Blacks, for reasons that are unclear. This may lead Black patients to receive less care because they are perceived as “lower risk,” although there is no evidence this is true.
- The Kidney Donor Risk Index incorporates race into the risk that a kidney will fail. The algorithm deems kidneys from Black donors as more likely to fail, based on previous cases of kidney transplants. This results in Black people being categorized as less suitable donors, which means longer waits for Black patients who are more likely to get kidneys from other Black donors.
- The Vaginal Birth after Cesarean algorithm labels Black and Hispanic women as less likely to have vaginal delivery after c-section. Race was included in the algorithm even though other variables such as insurance type were not included. That means that Black and Hispanic women are being steered toward c-sections, which puts them at a higher rate of complications.
- An algorithm to assess the risk of kidney stones labels Black people as having lower risk of a kidney stone, even though there is no evidence to show that Black people are less likely to have a kidney stone compared to other patients presenting with flank pain in the ED. This race adjustment could lead to misdiagnosis.
For most of these algorithms, there is no evidence to support including race in the algorithm. But even if race does correlate with clinical outcomes, does that mean it should be included? No, because differences in clinical outcomes based on racial group are usually due to social factors, not genetic ones.
“The racial differences found in large data sets most likely often reflect effects of racism,” the authors write. “In such cases, race adjustment would do nothing to address the cause of the disparity. Instead, if adjustments deter clinicians from offering clinical services to certain patients, they risk baking inequity into the system.”
Calling out and questioning racial adjustments in “evidence-based” clinical algorithms is an important step toward reducing health disparities.